Identifying Lung Cancer Using CT Scan Images Based On Artificial Intelligence
Abstract
Lung cancer appears to be the common reason behind the death of human beings at some stage on the planet. Early detection of lung cancers can growth the possibility of survival amongst human beings. The preferred 5-years survival rate for lung most cancers sufferers will increase from 16% to 50% if the disease is detected in time. Although computerized tomography (CT) is frequently more efficient than X-ray. However, the problem regarded to merge way to time constraints in detecting this lung cancer concerning the numerous diagnosing strategies used. Hence, a lung cancer detection system that usage of image processing is hired to categorize lung cancer in CT images. In image processing procedures, procedures like image pre-processing, segmentation, and have extraction are mentioned intimately. This paper is pointing to set off the extra precise comes approximately through making use of distinctive improve and department procedures. In this proposal paper, the proposed method is built in some filter and segmentation that pre-process the data and classify the trained data. After the classification and trained WONN-MLB method is used to reduce the time complexity of finding result. Therefore, our research goal is to get the maximum result of lung cancer detection.
Full Text:
PDFReferences
Sharma, S. (2018). A two-stage hybrid ensemble classifier-based diagnostic tool for chronic kidney disease diagnosis using optimally selected reduced feature set. International Journal of Intelligent Systems and Applications in Engineering, 6(2), 113-122.
Podolsky, M. D., Barchuk, A. A., Kuznetcov, V. I., Gusarova, N. F., Gaidukov, V. S., & Tarakanov, S. A. (2016). Evaluation of machine learning algorithm utilization for lung cancer classification based on gene expression levels. Asian Pacific journal of cancer prevention, 17(2),835-838.
Gindi, A., Attiatalla, T. A., & Sami, M. M. (2014). A comparative study for comparing two feature extraction methods and two classifiers in the classification of early-stage lung cancer diagnosis of chest x-ray images. Journal of American Science, 10(6), 13-22.
Suzuki, K., Kusumoto, M., Watanabe, S. I., Tsuchiya, R., & Asamura, H. (2006). Radiologic classification of small adenocarcinoma of the lung: radiologic-pathologic correlation and its prognostic impact. The Annals of thoracic surgery, 81(2), 413-419.
Aggarwal, T., Furqan, A., & Kalra, K. (2015, August). Feature extraction and LDA-based classification of lung nodules in chest CT scan images. In 2015 International Conference on Advances in Computing, Communications, and Informatics (ICACCI) (pp. 1189-1193). IEEE.
Jin, X. Y., Zhang, Y. C., & Jin, Q. L. (2016, December). Pulmonary nodule detection based on CT images using convolution neural network. In 2016 9th International symposium on computational intelligence and design (ISCID) (Vol. 1, pp. 202-204). IEEE.
Maurer, A. (2021). An Early Prediction of Lung Cancer using CT Scan Images. Journal of Computing and Natural Science, 39-44.
[8] Ignatious, S., & Joseph, R. (2015, April). Computer-aided lung cancer detection system. In 2015 Global Conference on Communication Technologies (GCCT) (pp. 555-558). IEEE.
Ghorai, S., Mukherjee, A., Sengupta, S., & Dutta, P. K. (2010). Cancer classification from gene expression data by NPPC ensemble. IEEE/ACM Transactions on Computational Biology and Bioinformatics, 8(3), 659-671.
Li, K., Liu, K., Zhong, Y., Liang, M., Qin, P., Li, H., & Liu, X. (2021). Assessing the predictive accuracy of lung cancer, metastases, and benign lesions using an artificial intelligence-driven computer aided diagnosis system. Quantitative Imaging in Medicine and Surgery, 11(8), 3629.
Das, R., & Sengur, A. (2010). Evaluation of ensemble methods for diagnosing valvular heart disease. Expert Systems with Applications, 37(7), 5110-5115.
Costa, V. S., Farias, A. D. S., Bedregal, B., Santiago, R. H., & Canuto, A. M. D. P. (2018). Combining multiple algorithms in classifier ensembles using generalized mixture functions. Neurocomputing, 313, 402-414.
Dande, P., & Samant, P. (2018). Acquaintance to artificial neural networks and use of artificial intelligence as a diagnostic tool for tuberculosis: a review. Tuberculosis, 108, 1-9.
Obulesu, O., Kallam, S., Dhiman, G., Patan, R., Kadiyala, R., Raparthi, Y., & Kautish, S. (2021). Adaptive diagnosis of lung cancer by deep learning Classification Using Wilcoxon gain and generator. Journal of Healthcare Engineering, 2021.
Khosravi, P., Kazemi, E., Imielinski, M., Elemento, O., & Hajirasouliha, I. (2018). Deep convolutional neural networks enable the discrimination of heterogeneous digital pathology images. EBioMedicine, 27, 317-328.
DOI: https://doi.org/10.29040/ijcis.v3i1.64
Article Metrics
Abstract view : 760 timesPDF - 308 times
Refbacks
- There are currently no refbacks.

This work is licensed under a Creative Commons Attribution 4.0 International License











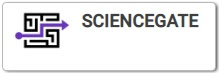




