Design of 3-Dimensional Simulation in Marching Drill Multimedia Based for Junior High School Students
Abstract
With multimedia-based technology, this is one area that is commonly used in learning methods. Computers also provide 3-dimensional (3D) technology which can become a new interest for students in the teaching and learning process. One of them is Marching Training (PBB). This application discusses marching, the benefits and objectives and regulations of marching. Therefore, a tool is needed to help learn commands and marching rules. So that a child can more quickly capture and understand what is conveyed through an interesting picture. In this way, students' interest in learning can be more motivated and can increase children's knowledge in using computers. Design is a creative activity towards something new and useful that did not exist before. According to Al-Bahra (2005: 51), design is the ability to create several alternative problem solutions. Meanwhile, according to Azhar Susanto (2004: 332), design is a general and detailed specification of computer-based problem solving that has been selected during the analysis stage.
Full Text:
PDFReferences
Adriyanto Bambang, 2010, Making Animation with Macromedia Flash 8, Center for Educational Information and Communication Technology, Ministry of National Education, 2010.
Anditya, Tanjung Syukri, 2015, Designing ArchiCAD & 3d Max House Designs, CV Andi Offset (Andi Publisher), Yogyakarta.
Firmansyah Zuli Agus Firmansyah, 2015, Official Scout Guide, Satyaku Kudarmakan, Darmaku Kubaktika, CV Wahyu Media, Jakarta.
Galih Pranomo, 2010, Studio MAX 2010 Basics and Applications, Andi Offset, Yogyakarta.
Hendratman Hendi, 2014, The Magic of Studio Max, Informatika-Bandung. Wahana Computer, 2010, Architectural Design Using AutoCAD and 3D Max 2010,CV Andi Offset (Andi Publisher), Yogyakarta.
Sarkonah, 2013, Enforcement Pocket Book, CV Nuansa Aulia, Bandung.
Ummah Khairul, 2014, We are Indonesian Scouts, PT. Masmedia Buana Pustaka, Sidoarjo.A. K. Fauziyyah, "Analisis sentimen pandemi Covid19 pada streaming Twitter dengan text mining Python", Jurnal Ilmiah SINUS, vol. 18, no.2, pp. 31-42, 2020, DOI : http://dx.doi.org/10.30646/sinus.v18i2.491
S. Symeonidis, D. Effrosynidis, and A. Arampatzis, "A comparative evaluation of pre-processing techniques and their interactions for twitter sentiment analysis", Expert Systems with Applications, vol.110, pp. 298-310, 2018, DOI : https://doi.org/10.1016/j.eswa.2018.06.022.
N. Hafidz, and D. Y. Liliana, "Klasifikasi Sentimen pada Twitter Terhadap WHO Terkait Covid-19 Menggunakan SVM, N-Gram, PSO", Jurnal RESTI (Rekayasa Sistem Dan Teknologi Informasi), vol.5, no.2, pp. 213-219, 2021, DOI : https://doi.org/10.29207/resti.v5i2.2960.
A. Salam, J. Zeniarja, and R. S. U. Khasanah, "Analisis Sentimen Data Komentar Sosial Media Facebook Dengan k-Nearest Neighbor (Studi Kasus Pada Akun Jasa Ekspedisi Barang J&T Ekspress Indonesia)". Proceeding of SINTAK, pp. 480 - 486, 2018.
G. Mostafa, I. Ahmed, and M.S. Junayed, "Investigation of different machine learning algorithms to determine human sentiment using Twitter data", International Journal of Information Technology and Computer Science, vol.13, no.2, pp. 38-48, 2021, DOI : 10.5815/ijitcs.2021.02.04.
E. R. Kalaivani, and E. R. Marivendan, "The Effect of Stop Word Removal and Stemming In Datapreprocessing", Annals of the Romanian Society for Cell Biology, vol.25, no.6, pp. 739-746, 2021.
E. Mulyani, F. P. B. Muhamad, and K. A. Cahyanto, "Pengaruh N-Gram terhadap Klasifikasi Buku menggunakan Ekstraksi dan Seleksi Fitur pada Multinomial Naïve Bayes", Jurnal Media Informatika Budidarma, vol.5, no.1, pp. 264-272, 2021, DOI : http://dx.doi.org/10.30865/mib.v5i1.2672.
F. Z. Ruskanda, "Study on the effect of preprocessing methods for spam email detection", Indonesia Journal on Computing (Indo-JC), vol.4, no.1, pp. 109-118, 2019, DOI : https://doi.org/10.21108/INDOJC.2019.4.1.284.
A. Deolika, K. Kusrini, and E.T. Luthfi, "Analisis Pembobotan Kata Pada Klasifikasi Text Mining", (JurTI) Jurnal Teknologi Informasi, vol.3, no.2, pp. 179-184, 2019.
B. J. Sowmya, C. S. Nikhil Jain, S. Seema, and S. KG, "Fake News Detection using LSTM Neural Network Augmented with SGD Classifier", Solid State Technology, vol.63, no.6, pp. 6985-9665, 2020.
F. T. Admojo, and Y. I. Sulistya, "Analisis performa algoritma Stochastic Gradient Descent (SGD) dalam mengklasifikasi tahu berformalin", Indonesian Journal of Data and Science, 3(1), pp. 1-8, 2022, DOI : https://doi.org/10.56705/ijodas.v3i1.42.
A. Tuomo, J. Suutala, J. Röning, and H. Koskimäki, "Better classifier calibration for small datasets", ACM Transactions on Knowledge Discovery from Data (TKDD), vol. 14, no.3, pp. 1-19, 2020, DOI : https://doi.org/10.1145/3385656.
F. Pedregosa, G. Varoquaux, A. Gramfort, V. Michel, B. Thirion, O. Grisel, ... and E. Duchesnay, "Scikit-learn: Machine learning in Python", the Journal of Machine Learning research, vol.12, pp. 2825-2830, 2011.
J. Xu, Y. Zhang, and D. Miao, "Three-way confusion matrix for classification: A measure driven view". Information sciences, vol.507, pp. 772-794, 2020, DOI : https://doi.org/10.1016/j.ins.2019.06.064.J.
DOI: https://doi.org/10.29040/ijcis.v4i4.140
Article Metrics
Abstract view : 200 timesPDF - 140 times
Refbacks
- There are currently no refbacks.

This work is licensed under a Creative Commons Attribution 4.0 International License











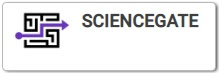




