Performance Testing of KNN and Logistic Regression Algorithms in Classifying Heart Disease Susceptibility
Abstract
The annual global death toll due to cardiovascular diseases, which fall into the category of heart and blood vessel disorders, reaches 17.9 million lives. This undoubtedly requires more attention in order to anticipate the potential risk of heart attacks that can affect anyone at any time. Data analysis or data mining approaches have become a significant contribution in the field of information technology to provide valuable information regarding the risk of heart diseases. Data analysis using the K-Nearest Neighbor and Logistic Regression algorithms is expected to provide information related to the susceptibility category for heart diseases, such as age susceptibility, gender, cholesterol levels, and so on. With the information obtained from this data analysis, it is hoped that it can serve as a reference and consideration for individuals to be more vigilant in maintaining their health. The results indicate that the highest correlation with susceptibility to heart disease is based on a person's age and their body weight. The correlation coefficient between these two variables is 0.37, suggesting a relationship between a person's age and their body weight, which can make them more susceptible to heart disease. Testing with both algorithms shows a high level of accuracy, with K-Nearest Neighbor achieving an accuracy rate of 0.95, while Logistic Regression has an accuracy of 0.96.
Full Text:
PDFReferences
A. F. D. Zakha MaisatEka Darmawana, ”Implementasi Optimasi Hyperparameter GridSearchCV Pada Sistem Prediksi Serangan Jantung Menggunakan SVM,” Teknologi: Jurnal Ilmiah Sistem Informasi, pp. 8-15, 2023.
A. F. P. Novanto Yudistira, ”Algoritma Decision Tree Dan Smote Untuk Klasifikasi Serangan Jantung Miokarditis Yang Imbalance,” Jurnal Litbang Edusaintech, vol. 2, nr 2, pp. 112-122, 2021.
S. S. N. L. Z. M. &. C. X. N. Satish Chandra Reddy, ”Classification and Feature Selection Approaches byMachine Learning Techniques: Heart Disease Prediction,” International JournalofInnovative Computing , vol. 9, nr 1, pp. 39-46, 2019.
M. P. a. S. Parija, ”Prediction of Heart Diseases using Random Forest,” Journal of Physics: Conference Series, vol. 1817, nr 1, p. 012009, 2021.
N. T. S. T. a. B. T. Nguyen, ”Intelligent Information and Database Systems,” i 9th Asian Conference, ACIIDS 2017, Kanazawa, Japan, 2017.
B. W. E. a. D. T. Sherrill, ”Analysis of Student Data for Retention Using Data Mining Techniques.,” i 7th Annual National Symposium on Student Retention, Oklahoma, 2011.
Suyanto, Data Mining untuk klasifikasi dan klasterisasi data., Bandung: Informatika Bandung , 2017.
J. C. Y. H. &. I. C. Jacob Benesty, Noise Reduction in Speech Processing : Pearson Correlation Coefficien, Springer, 2009.
L. Wang, ”Research and implementation of machine learning classifier based on KNN.,” i IOP Conference Series: Materials Science and Engineering, IOP Publishing, 2019.
R. a. K. P. Ewing, Basic quantitative research methods for urban planners, London: Routledge, 2020.
DOI: https://doi.org/10.29040/ijcis.v4i4.133
Article Metrics
Abstract view : 287 timesPDF - 162 times
Refbacks
- There are currently no refbacks.

This work is licensed under a Creative Commons Attribution 4.0 International License











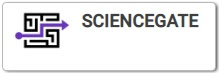




