Texture Characteristic of Local Binary Pattern on Face Recognition with Probabilistic Linear Discriminant Analysis
Abstract
Face recognition is an identification system that uses the characteristics of a person's face for processing. There is a feature in the face image so that it can be distinguished between one face and another face. One way to recognize face images is to analyze the texture of the face image. Texture analysis generally requires a feature extraction process. In different images, the characteristics will also differ. This characteristic will be the basis for the recognition of facial images. However, existing face recognition methods experience efficiency problems and rely heavily on the extraction of the right features. This study aims to study the texture characteristics of the extraction results using the Local Binary Pattern (LBP) method which is applied to deal with the introduction of Probabilistic Linear Discriminant Analysis (PLDA). The data used in this study are human face images from the AR Faces database, consisting of 136 objects (76 men and 60 women), each of which has 7 types of images Based on the results of testing shows the LBP method can produce the highest accuracy with a value of 95.53% in the introduction of PLDA.
Full Text:
PDFReferences
1. Hamouchene, I., Aouat, S., dan Lacheheb, H., 2014, Texture Segmentation and Matching Using LBP Operator and GLCM Matrix, In Intelligent Systems for Science and Information, Springer, Algeria, hal. 389–390.
2. Arriawati, A. J., Santoso, I., dan Christyono, Y., 2011, Pengenalan Citra Tekstur Menggunakan K-Nearest Neighbour Berdasarkan Ekstraksi Ciri Metode Matriks Kookurensi,. Jurusan Teknik Elektro, Fakultas Teknik, Universitas Diponegoro, Semarang.
3. Hidayat, R., 2015, Studi Komparasi Metode Ekstrasi Ciri Wajah Untuk Pengenalan Ekspresi Wajah Manusia Berbasis Pengolahan Citra Digital,. Universitas Gadjah Mada, Yogyakarta.
4. Wibowo, M. E., Tjondronegoro, D., dan Zang, L., 2012, On the application of the probabilistic linear discriminant analysis to face recognition across expression, In IEEE International Conference on Multimedia and Expo Workshops (ICMEW ), Melbourne, hal. 459–464.
5. Maturana, D., Mery, D., dan Soto, Á., 2010, Face recognition with local binary patterns, spatial pyramid histograms and naive bayes nearest neighbor classification, In Proceedings - International Conference of the Chilean Computer Science Society, SCCC, Chile, hal. 125–132.
6. Ojala, T., Pietikainen, M., dan Harwood, D., 1996, A comparative study of texture measures with classification based on feature distributions, Pattern Recognition, 29, 1, 51–59.
7. Rassem, T.H. dan Khoo, B.E., 2014, Completed Local Ternary Pattern for Rotation Invariant Texture Classification, The Scientific World Journal, 1–10.
8. El Shafey, L.E., 2014, Scalable Probabilistic Models for Face and Speaker Recognition,. Faculté des Sciences et Techniques de l’Ingénieur laboratoire de l’IDIAP, Lausanne,
9. Prince, S.J.D. dan Elder, J.H., 2007, Probabilistic Linear Discriminant Analysis for Inferences About Identity,
10. Wibowo, M. E., Tjondronegoro, D., dan Zang, L., 2012, On the application of the probabilistic linear discriminant analysis to face recognition across expression, In IEEE International Conference on Multimedia and Expo Workshops (ICMEW ), Melbourne, hal. 459–464.
11. Shaikh, M.K., Tahir, M.A., dan Bouridane, A., 2014, PROBABILISTIC LINEAR DISCRIMINANT ANALYSIS FOR INTERMODALITY FACE RECOGNITION, In IEEE International Conference on Acoustic, Speech and Signal Processing (ICASSP), hal. 509–513.,
12. Li, P., Fu, Y., Mohammed, U., Elder, J.H., dan Prince, S.J., 2012, Probabilistic Models for Inference about Identity, IEEE Transactions on Pattern Analysis and Machine Intelligence, 34, 1, 144–157.
DOI: https://doi.org/10.29040/ijcis.v1i1.10
Article Metrics
Abstract view : 712 timesPDF - 489 times
Refbacks
- There are currently no refbacks.

This work is licensed under a Creative Commons Attribution 4.0 International License











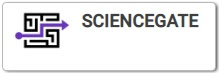




